In a prospective, cross-sectional study, researchers from Cincinnati Children's Hospital Medical Center found that ultrasound-derived fat projection (UDFF) yielded 94.1% sensitivity for detection of MRI proton-density fat fraction (PDFF) measurements exceeding a commonly used threshold for clinically significant hepatic steatosis.
"These results support a clinical role of UDFF in the detection of hepatic steatosis, with a UDFF > 5% having high sensitivity for detection of MRI PDFF ≥ 5.5%," wrote corresponding author Dr. Andrew Trout and colleagues.
Although increased hepatic echogenicity and associated decreased conspicuity of the portal triads on ultrasound has been utilized to identify hepatic steatosis, the modality has -- unlike MRI -- not traditionally been able to provide true quantification of liver fat. In their study, the researchers sought to evaluate the performance of UDFF, one of several new methods developed by ultrasound vendors for detecting and quantifying hepatic steatosis on ultrasound.
They included 56 overweight and obese adults and adolescents ≥ 16 years in the study. During a single visit between August and October 2020, all participants received investigational liver MRI on a Signa Architect 3-tesla MRI scanner (GE Healthcare), as well as an ultrasound exam with a DAX deep abdominal transducer on an Acuson Sequoia ultrasound scanner (Siemens Healthineers).
UDFF, a clinically available technique developed by Siemens, was measured on all patients. The method is derived from the measurement and combination of both attenuation effect and backscatter. After a median UDFF is calculated across five measurements on all three acquisitions, a final UDFF percentage is then produced based on the median of the three acquisitions, according to the researchers.
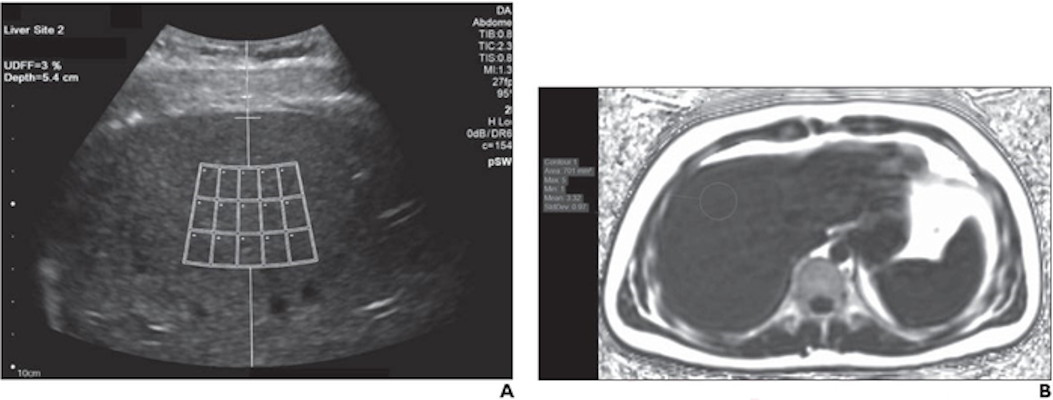
In the study, three MRI PDFF acquisitions were also completed using GE's Ideal IQ whole-liver 3D axial confounder-corrected technique. An image analyst then calculated the PDFF measurements under the supervision of a pediatric radiologist with 10 years of post-training experience, according to the researchers.
The UDFF measurements had a positive association with MRI PDFF results, producing a rho of 0.82 and intraclass correlation coefficient of 0.84. The mean bias between UDFF and PDFF was 4%.
Overall, UDFF had an area under the curve of 0.9 for diagnosing MRI PDFF ≥ 5.5 -- the threshold considered to represent clinically significant hepatic steatosis. A UDFF overall cutoff of > 5% produced 94.1% sensitivity and 63.6% specificity for diagnosing MRI PDFF ≥ 5.5.
The researchers noted that liver UDFF is expressed as a percentage, analogous to MRI PDFF.
"Thus, not only is diagnostic performance for a specific threshold fat fraction clinically relevant, but the correspondence in measured fat fraction percentage between techniques is also relevant, particularly if the techniques are used to monitor for change in fat fraction over time or in response to therapy," they wrote.
Furthermore, the area under the receiver operating characteristic curve for UDFF did not differ significantly based on the number of measurements. As a result, the researchers concluded that three measurements are likely sufficient for determining UDFF in clinical practice, according to the researchers.
"Use of three UDFF measurements is anticipated to achieve similar results as obtained from a greater number of measurements while improving examination efficiency; we do not advise use of a single measurement given the possibility of obtaining a single erroneous value," they wrote.